When we think of basketball data analytics, it’s often linked to pre-game or post-game analysis for coaching staff. However, basketball data analytics has much broader applications that can be invaluable for player representation agencies. Let's break down how data analytics can be a game-changer for agencies.
Talent Identification:
Data analytics is a powerful tool for spotting emerging talents or those who might fly under the radar. It’s crucial when it comes to recruiting new players. By exploiting data, agencies can filter a large pool of players, narrowing down the field to focus more intensively on a select few who show the most promise.Supporting Intermediation:
When intermediating with a club, an agency can present players who fit the coach’s preferences and back up their needs with advanced analytics. This data-driven approach can provide a solid foundation for discussions, making the agency’s proposals more compelling.Market Value Justification:
Data can be used to justify a player’s market value during negotiations. By presenting a detailed, data-backed case, agencies can ensure that their players are valued appropriately, reflecting their true worth in the market.Player Comparison:
Agencies can utilize data to compare the performance of their players against competitors. This comparative analysis can highlight the strengths and potential of their players, providing a strategic advantage in negotiations and positioning.
For agencies looking to incorporate data as a core tool in their operations, using data platforms like Hudl Instat offers a fast and efficient solution. Hudl Instat provides access to data from various leagues on a single platform, making it easy and quick to use.
With tools like Hudl Instat, you don’t need to be a data scientist to make data a fundamental part of your agency’s strategy. For more complex analyses, services like those offered by Basketball Analytics Lab can be the solution.
Practical Example
For instance, after a standout performance in the Olympics, Guerschon Yabusele decides to move to the NBA. Among the players in an agency’s roster, the one who most closely resembles Yabsuele in playing style (shot types, efficiencies, rebound percentages, etc.) can be identified. Additionally, the agency can pinpoint the players who would be agencies competition. This knowledge can give a significant advantage in negotiations.
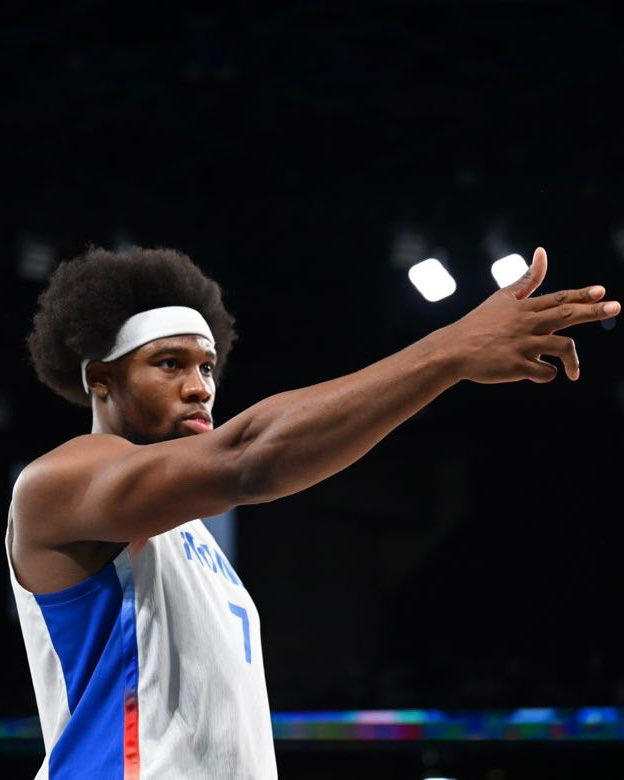
Let's explore this scenario from three perspectives:
Method 1
The comparison process can be done directly through the Hudl Instat platform. The first step would be to identify Yabusele’s primary types of finishes. In this case, we've analyzed data from the 2023-24 season.
After identifying Yabusele’s key finishes, we can compare different players. Here, we compare him with Davis Bertans, rumored to be Yabusele’s potential replacement.
In the first column, we have Yabusele’s data (2023-24 season), the second column shows Bertans’ NBA data (2023-24 season), and the third column contains Bertans’ data from his 2015-16 ACB and Euroleague season (though some games are missing). This straightforward method allows agencies to assess how various players in their roster might fit into Yabusele’s role.
Method 2
In this analysis method, we can compare all the players in a league and rank them based on the metrics we choose in the Hudl Instat platform. For example, we’ve analyzed Euroleague players from the 2023-24 season based on their Catch & Shoot made percentage (considering only those with more than 1.6 Catch & Shoot attempts per game). The top 5 players we identified are:
Nikos Rogkavopoulos
Billy Ouattara
Alec Peters
Semi Ojeleye
Ioannis Papapetrou
From this list, we’d need to remove any players who don’t match Yabusele’s position. Once filtered, we can compare the selected players with Yabusele, just as we did in Method 1. Although we’ve used Euroleague players for this example, the same approach can be applied to various leagues.
Using this method, agencies can identify which players might pose the most competition.
Method 3
Beyond direct comparison using the Hudl Instat platform, a more advanced analysis can be performed with a solid understanding of data analytics. Data can be downloaded from Hudl Instat in Excel format, allowing for further manipulation. This process involves clustering and calculating similarity scores to identify players most like Yabusele. Here's how the process works:
Download player data from the Euroleague and Eurocup for the 2023-24 season.
Group all players into nine groups using k-means clustering. In this case, we’ve used play type metrics to classify players according to their tendencies.
After clustering, we can identify players within Yabusele’s cluster who have similar finishing tendencies. From now on we work only with players from the Yabusele cluster.
Next, we analyze shooting efficiencies for the most frequently used play types and calculate a similarity score. This score reflects how closely one player’s data matches another’s, with a value of 1 indicating identical data. This is done by calculating the Euclidean distance.
The players with a similarity score greater than 0.8 compared to Yabusele are:
Again, we must consider the position of each player, as some may not fit Yabusele’s role. However, this method can identify players who might otherwise fly under the radar and provides a quantitative reference that adds perspective to player rankings.
This technique requires advanced knowledge but can offer a significant competitive edge over other agencies.
At Basketball Analytics Lab, we offer data analytics services to clubs, enabling them to enhance their performance without significantly increasing their budgets.
Feel free to share and comment if you found this article interesting.
Where Data Meets the Hoops - Let's Analyse the Game Together!